Dr. Bessie Noll, Post Doctoral Researcher, Energy and Technology Policy Group, ETH Zürich
To address global sustainability challenges, the role of policy intervention in accelerating technological change is crucial. While most policy interventions occur on the local level, their innovation effects can spill over to other jurisdictions, potentially having global impact. Our recent paper (Noll et al. 2023) presents a comprehensive modeling framework that integrates endogenous technological learning using experience curves and deployment spillovers to project future technological costs and deployment. This framework is pivotal for understanding the impacts of local policy interventions on global technological transitions, taking the road-freight sector as a case study. Below are short descriptions of key aspects of this framework and the relevance of the research for the larger Integrated Assessment Model (IAM) community.
Endogenous Technological Learning and Experience Curves
Technological learning, represented through experience curves, captures how technology costs decline with cumulative deployment. By endogenizing this relationship into technology competition models, we can better project how policies and market dynamics influence technological change. The system dynamics model presented in this research article simulates competition between incumbent and emerging technologies, factoring in learning rates to project cost trajectories and market shares.
Global vs. Local Learning
A key aspect of the study is the differentiation between global and local learning effects. Global learning refers to the broader, cumulative knowledge and cost reductions experienced worldwide as technologies are deployed. Local learning, on the other hand, is the specific knowledge and cost reductions that occur within a particular region or market. Our model demonstrates how local interventions can catalyze significant global learning benefits for standardized technologies that are globally produced and traded. For example, strong local policies in major markets can not only reduce costs within those markets but also contribute to global cost reductions through shared knowledge and increased production scales.
Modeling Deployment Spillovers
Our framework incorporates global learning effects by including deployment spillovers across applications and regions. Local policy interventions can have far-reaching effects, promoting technological adoption beyond their initial jurisdiction. Our model quantifies these spillovers, demonstrating how strong public interventions in large or multiple regions can significantly accelerate the global transition to low-carbon technologies.
Application to Commercial Road Freight Sector
The road-freight sector, responsible for nearly 13% of global greenhouse gas emissions, serves as an ideal case study. Though low-carbon (and less air-polluting) alternative technologies for road freight have emerged, uncertainty remains around which technologies to transition to, how fast, and in which specific applications. Our model reveals that electric vehicles (EVs) in road freight can grow significantly, especially with robust local policy support. For instance, interventions in major markets like China (see Figure 1) and the European Union can drive global EV adoption through spillover effects.
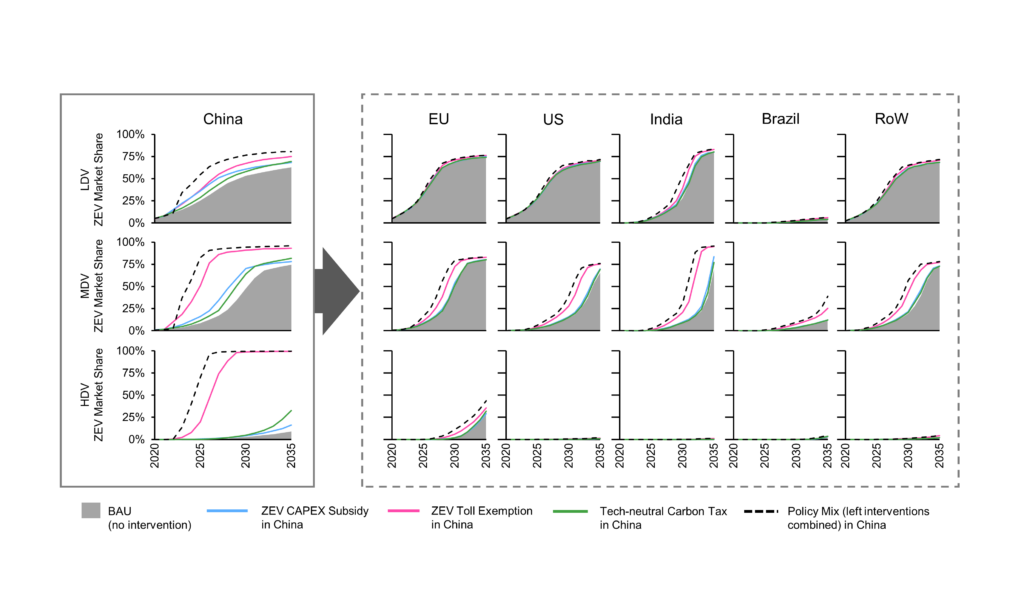
Figure 1 . Model projection results showing zero-emission vehicle (ZEV) market shares in all modelled regions under different policy intervention scenarios in China. ZEV market shares in China on the left are a direct result of local interventions. Increased ZEV market shares in all other regions to the right are an indirect result of spillovers.
Relevance for Integrated Assessment Models (IAMs)
Our findings are highly relevant for larger IAMs, which guide global climate policy. By incorporating endogenous learning effects, differentiating local vs. global learning rates, and inclusion of deployment spillover effects, IAMs can provide more accurate projections of technological change and thus derive important policy recommendations. While IAMs may include resolution in different countries or world regions, technology costs are typically global parameters, following a global learning curve. Examining “local” intervention spillover effects in IAMs is therefore difficult. The research presented in our paper offers a framework and starting point for extending these kinds of features in IAMs.
In conclusion, this research underscores the importance of policy-driven technological change and the role of deployment spillovers in achieving global sustainability. It provides a robust tool for policymakers and researchers to design effective strategies for decarbonizing critical high-carbon sectors.
This news is part of a project that has received funding from the European Union’s Horizon Europe programme under grant agreement No 101081604 – PRISMA.
Views and opinions expressed are however those of the speaker(s) only and do not necessarily reflect those of the European Union or the European Climate, Infrastructure and Environment Executive Agency (CINEA). Neither the European Union nor the granting authority can be held responsible for them.